Czy 500+ zniechęca kobiety do pracy? Odpowiedzi szukamy z Filipem Premikiem w najnowszym odcinku podcastu GRAPE | Tłoczone z danych.
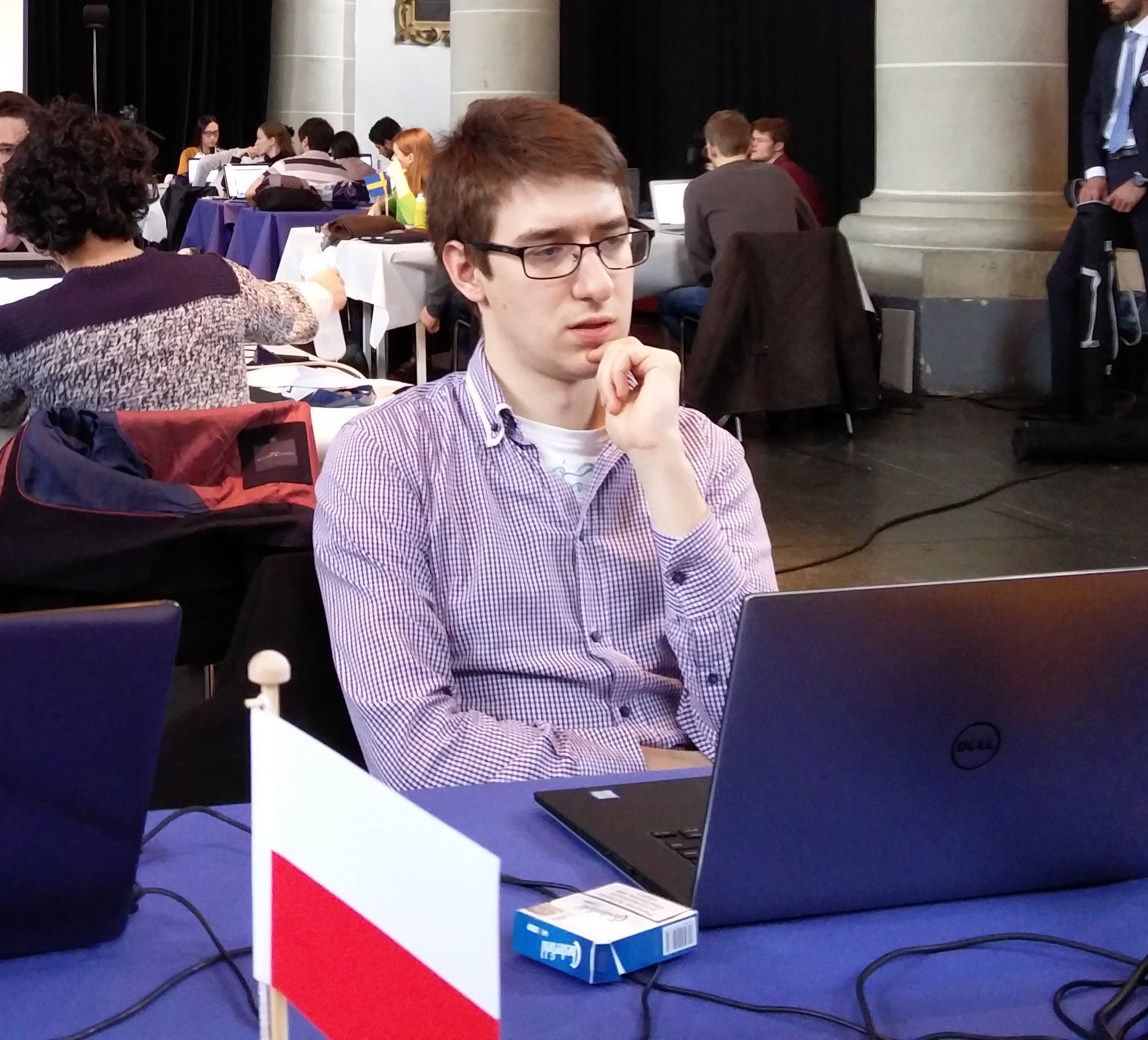
Filip
Premik
Filip follows a PhD program at University of Minnesota and does research at GRAPE.
Filip realizuje program doktorski na University of Minnesota i prowadzi badania w GRAPE.
-
-
Co by było gdyby? O przyczynowości w nauce, na przykładzie 500+, pisze Filip Premik GRAPE | Tłoczone z danych.
-
Rozmowa z Katarzyną Mokrzycką w 300Gospodarka.pl
Opublikowane | Published
-
Evaluating the 500+ child support program in Poland | Gospodarka Narodowa Przeczytaj streszczenie | Read abstract
We investigate immediate effects of a large scale child benefit program introduction on labor supply of the household members in Poland. Due to nonrandom eligibility and universal character of the program standard evaluation estimators are likely to be inconsistent. In order to address this issues we propose a novel approach which combines difference-in-difference (DID) propensity score based methods with covariate balancing propensity score (CBPS) by Imai and Ratkovic (2014). The DID part solves potential problems with non-parallel outcome dynamics in treated and non-treated subpopulations resulting from non-experimental character of the data, whereas CBPS is expected to reduce significantly bias from the systematic differences between treated and untreated subpopulations. We account also for potential heterogeneity among households by estimating a range of local average treatment effects which jointly provide a reliable view on the overall impact. We found that the program has a minor impact on the labor supply in periods following its introduction. There is an evidence for a small encouraging effect on hours worked by treated mothers of children at school age, both sole and married. Additionally, the program may influence the intra-household division of duties among parents of the youngest children as suggested by simultaneous slight decline in participating mothers' probability of working and a small increase in treated fathers' hours worked.
W toku | Work in progress
-
Estimating the effects of universal transfers: new ML approach and application to labor supply reaction to child benefits Przeczytaj streszczenie | Read abstract
This paper evaluates effects of introduction of a universal child benefit program on female labor supply. Large scale government interventions affect economic outcomes through different channels of various magnitude and direction of the effects. In order to account for this feature, I develop a model in which a woman decides whether to participate in the labor market in a given period. I show how to use the resulting decision rules to explain flows in aggregate labor supply and simulate counterfactual paths of labor force. My framework combines flexibility of reduced form approaches with an appealing structure of dynamic discrete choice models. The model is estimated nonparametrically using recent advances in machine learning methods. The results indicate a 2-4 percentage points drop in labor force among the eligible females, mainly driven by changes in women's perceived trade-offs and beliefs that discouraged inflows.
In addition to this study, I also present a variety of sensitivity analyses. With the development of statistical theory behind the machine learning algorithms, they are becoming an important tool in the empirical economists' toolbox. By construction, they rely on a set of pre-specified hyper parameters governing the architecture of the algorithm chosen arbitrarily by a researcher. In this note, I show that the economic interpretation of the estimates (obtained via Generalized Random Forest by Susane Athey, Julie Tibshirani, and Stefan Wager ) is robust to different choices of the hyper parameters. This is an encouraging result suggesting that despite their complexity, the machine learning algorithms are likely to become a part of applied econometricians' toolbox.